Editor’s note: Investor and entrepreneur David Gardner is founder of Cofounders Capital in Cary and is a regular contributor to WRAL TechWire. He and other columnists are part of our regular Startup Monday package.
+++
CARY – There has been a tremendous amount of attention and VC investment dollars flowing into ChatGPT, Bard, Galactica and other Large Language Model (LLM) AIs of late with amazingly impressive and disappointing results. AIs can now pass the medical board exam and instantly write insightful essays in any style or persuasion requested but there have been serious problems with accuracy. The Bard and Galactica rollouts, for example, were quickly pulled back for more testing.
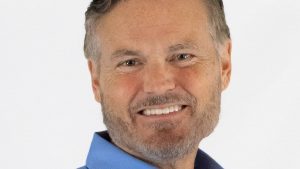
David Gardner (Cofounders Capital photo)
I view these new AI tools like child savants. They can be brilliant and totally lack common sense at the same time. One AI wrote a convincing article on the benefits of putting wood chips in your salad and ground up glass in breast milk.
It’s all about the training data
It wasn’t that long ago that AI stock picking engines were all the rage. Because they were trained on historical data, when tested, the outcomes they predicted from any point in the past were rather accurate. But the world continues to change and investors soon learned that historical data is not always the best predictor of future results.
LLMs today can analyze millions of articles, studies and reports, find patterns and write insightful answers to questions using that data. But the AIs will be no better than the data they are training on. I shudder to think of some of the racist books, fake news and false Covid cures found all over the internet. Since LLMs don’t cite their sources, it is hard to determine what data is driving their conclusions.
I have searched extensively and can’t seem to find how various LLMs weigh the credibility of the millions of sources that influence their decision making. When queried about sources, Chat GPT responds with what appears to be a scripted response saying that the author’s credentials are considered. There does seem to be a consensus component i.e. what do most sources say? That really scares me because an ever increasing number of articles and reports on the internet are created not for accuracy but rather for a marketing or propaganda purpose. The old saying, “garbage in leads to garbage out” comes to mind. Will the prejudices, greed and self-serving motives of our most prolific be the stuff that trains our AIs in the future? This is important because more than likely, AIs may be doing much of the training of our children in the future. It’s already doing some of their homework!
AI is here to stay and becoming increasingly important
Even with my major concerns about LLMs, I use them a lot. ChatGPT, for example, generates an excellent list of competitive companies when I’m doing diligence on a potential investment. Its ability to recognize patterns is revolutionary in fields like radiology and gene-related life sciences research. Many fear it will replace human workers but I think supplementing them is more likely. I won’t be replacing my attorney for ChatGPT but I may be replacing an attorney who does not use ChatGPT for one that does!
We are not investing in Chat GPT or any LLM-based ventures today
So, what does all of this mean for early stage investing? The big VC funds are putting a lot of money into LLMs but keep in mind that they are investing in the core science. You need to be able to write very large checks to fund a science project through commercialization. This makes sense for the largest funds but not for us early stage investors. I remember back in the 90s when everyone was losing money on their dot bomb investments, those big funds that had invested in infrastructure such as data farms and fiber, etc. did OK.
There is no doubt that once it has matured, there will be many viable LLM business models and applications for early stage investors. One of the larger concerns we have today with LLM-based ventures is pricing. As my friend and mentor, Alex Osadzinski keeps reminding me, we don’t yet know what Microsoft, Google and others will charge for these tools. I see single transaction cost, CPU and bandwidth alone, at over 4 cents per query. Some LMMs are trying to control their costs by answering easier queries using smaller data sets but with accuracy already a problem the future of this approach is uncertain. As for now, these tools are still in free-beta. How can we model a new venture when we don’t even know what their transactional price is going to cost?
Conclusion
I still think Bill Gates was right when he wrote in his first book how we tend to overestimate the effects that new technology will have for the first five years and underestimate its impact the following five years. Venture investing is a long game. There will be time for us early stage investors to deploy capital in LLM enabled ventures but not yet. For now, it is enough for me that entrepreneurs have at least stopped saying “blockchain” in every presentation!